04: Text to Music: It is not that easy!
This is an automated AI transcript. Please forgive the mistakes!
Hello humans! Welcome to a new episode of the Eliak Suite. I've got some numbers
for you today in the beginning. The Digital Music Distribution Publishing and
Licensing Service Tune Core recently published a study about artificial intelligence
and music. Here are the results. Out of 1500 artists from over 20 countries,
the USA and France representing most of the participants, 30 % are aware and engaged
with AI. They rather think positively about it. 39 % rather have fears,
the rest is probably undecided. 27 % have used AI tools, most of them for creative
artwork. Around a third wants to use generative AI in the creative process.
Around 30 % will grant consent for their music, voice, recognition, and artwork
creation to be used in generative AI. But the majority of them would only do that
with a permission, compensation, and credits. More than 60 % of them fear being
replaced by AI -generated music, plagiarism, and fair distribution of recorded music
revenue. Last but not least, half of them show willingness to offer them music for
machine learning. But how would this data of music be used and and analyzed in
these datasets, we will talk about that today on this little show.
Und mein Name ist Dennis Kastrup.
♪ To the skies and sea ♪
♪ I'm just a poor boy ♪ ♪ I need no sympathy ♪ ♪ 'Cause I'm easy come,
easy go ♪ ♪ Little high, little low ♪ ♪ Anywhere the wind blows And love doesn't
really matter to me,
to me
My love, I just killed a man Put a gun against his head,
pull the mark trigger, now he's dead Mama,
life had just begun, and now I've gone and thrown it all away
Mama, Oh, didn't mean to make you cry If I'm not bad,
forget this time tomorrow Carry on, carry on Nothing really matters
It's too late, my time has come Since shivers down my spine Body's aching all the
time Goodbye, everybody I've got to go ♪ Leave it all behind and face the truth ♪
♪ My mouth ♪ ♪ I don't wanna die ♪ ♪ I sometimes wish I'd never been born at
all ♪ ♪ Carry on, carry on ♪ ♪ Nothing we matters
I
see a little shadow, a little other man Can we do the pandango,
thumb and ball to line him The river refriending me, canaleo,
Galileo, Galileo, Philo
Spare him his life from his monstrosity Mamma mia,
mamma mia, mamma mia, let him go Beelzebub has a devil put aside For me,
for me, for me
Oh,
so you think you can stop me and spit my eyes Oh,
so you think you can love me ♪ You need to die, oh,
baby ♪ ♪ Don't do this to me, baby ♪ ♪ Just gotta get out,
just gotta get right out of here ♪
♪ Anyone can see, nothing really matters,
nothing really matters to me.
Whitney Houston singing Bohemian Rhapsody by Queen. The user Alex on YouTube who
uploaded this video is definitely a Mariah Carey and Whitney Houston fan. All of his
or her videos are AI versions with the two voices of the singers.
This AI version of that famous hit does not have the original music in the
background, but a piano version of it. And this happens often with these kinds of
songs. Unfortunately I do not have any more information how it was made, like a lot
of times. This is what I think. He or she took an existing piano instrumental of
Bohemian Rhapsody and let someone sing it. A friend. Or is it her who did it by
herself? I also think it is always the same person singing in all of the videos,
because sometimes the voice breaks a bit and you can hear the original. I chose
this version because it is an impressive example of what is already possible. Also
impressive these days is music that is generated by an AI. There are Aiva,
Refusion and others. And since a couple of years also Moobard. Creating a voice
where you train on one singer is not that difficult anymore. Training on music in
general is really really difficult because first of all you have to be able to
describe music so that the model understands. About that I have talked with Paul
Skordan, CEO and head of music at Moobird. He explained to me what exactly they are
doing. Our users are mainly content creators and different artists who want to add
some sound track for their content, for the video reel or vlog or even NFT,
so they can visit our product, Weber Trender, and generate sound track there,
so they only have to put some text description in the special field and set the
duration of a track, so five minutes, up to 25 minutes,
choose a type of a track. We have different types for different occasions and like
game goal or mix or loop and hit generate and Mubert will generate some suitable
soundtrack with these settings, so if you choose text prompt,
this text prompt will transform into some kind of special data which we can use
with our generator, or user can choose a genre or a mood or activity and generate
a track with these settings without any text drums if they don't have an idea about
how this music can sound.
(upbeat music)
(upbeat music) So I searched for music that would fit the words of Paul in the
background. I actually did not have really a clue what I should search for, but I
gave it a try with the words, cool, fast beats with attitude.
Doesn't really make sense, but I think it works. Don't ask me how I got this idea.
That is the music you are listening to right now. I generated music trained on
music in the dataset but where does Mubart has its data from? Mubart works with a
dataset with around two and a half million of different sounds in different genres,
moods, like keys, scales, tempos. So we have this large base of different sounds.
And all of these sounds are tagged by different words. So with genres,
activities, moods, themes, like different tags, we have a complex tagging system.
And when user type in prompt in our special field,
this prompt goes to text transformer artificial intelligence that can transform this
prompt in our tags that connected with sounds.
So basically it's like a text prompt. It converts to some line of different tags
and we generate music from these corresponding sounds that have some tags from this
prompt. Okay, so let's recap. The songs are described with metadata, with tags.
But who does the work of tagging the music in the dataset? Musicians, because
musicians and sound designers are applauded in these sounds to our bass.
We have a special platform for artists, Mibert Studio. And we pay musicians for
these licenses, for these samples. So our data set is ethically sourced.
So because we have all agreements, all contracts with these musicians. So when they
upload these sounds, they can tag it or we can ask them to tag it.
Yeah, so if you want to get paid and upload some sounds,
we can ask you to tag it also.
Let's try this out! I asked Mubet to generate me something with the words "walking
the beach with a cocktail in your hand". For this prompt I think that it will
generate something suitable, but of course we don't have all music genres and all
moods in our dataset. So, Mubert will generate something that can generate,
but of course it can be not relevant at all, but it will try to guess what the
user needs. So, I think that it will choose our summer channel with some indie
house, with some light arrangement and funky beats,
So something like that, because it has something about summer, about beach,
about positive vibes. So it can find our tags that's relevant to this prompt.
Of course, these kind of AI -generated songs are scary for people who create music
that is used maybe in commercials, films or even podcasts like this one.
And I understand, and as mentioned here many times before, I hope we will find a
model soon that will help everyone in the future to get paid properly. But when
there is a bad side, there it also be a good site. Actually, it's like lowering
the barrier for entering the music world. So you maybe don't know how to play
instruments or how to set like equalizers, compressors and like tweak synthesizers,
but you can create something with your ideas. And Yeah,
so this kind of personalization of music, it's also like democratization and listeners
can become musicians in some way. So they can get some interest in this field and
maybe go to music school. So what do you think? AI generated music as a tool, evil
or amazing? Leave me a comment via mail, you find a link to my home page in the
notes of all the episodes. I'm curious what you think, but let's come back to the
tagging system, the metadata of songs and data sets, so the music with which an AI
was trained. I wanted to know a bit more about that, how the tagging of songs
works and I reached out to Roman Gebhardt, Chief Artificial Intelligence Officer of
Cyanide, a German company. So at Cyanide we offer AI solutions for basically everyone
that is working with music, can be labels, playlist created, production music
libraries, artists. We offer AI tools for analyzing music,
tagging music and also search algorithms, which can be sound -based search,
it can be free text search and any kind of different form of multimodal search
experiences.
So you type in words and The search engine finds in an archive the existing songs
with the help of an AI that has learned the descriptions of the songs and connect
them to other songs. The music you are hearing and we just heard before was exactly
found like this. In this special case I tried out Slip Point Stream, a service that
searches royalty free music in a catalogue and for that they use Cyanide's
technology. My search was pop and good vibes and the search engine spit out for me
next to other thousands of songs dance floor therapy by the artist mom bought let's
listen a bit more to this instrumental
What do you think? Does that sound like pop and good vibes? What I like a lot
about slipstream, you can use the music under special circumstances, which are
mentioned in the notes of a podcast, for example, where you downloaded it from and
what the name of the song is, the license link. Done. You can find all the songs
in the notes of this episode online next to all the other music I used. What I
also liked. If you are an artist and the service sees that you downloaded his or
her track, you get personal messages from him or her in which you are kindly asked
to support their music and give proper attributions. A very good idea in my opinion.
But to make it clear again, in context of the podcast, we are here not talking
about generative AI. It is just a search engine that is based on artificial
intelligence, that finds songs based on descriptions. But how do we describe music
the best anyhow? That could be moods, emotions, it could be genres, it could be
styles, it could be instruments and so on. This is all categories that we have,
which we automatically tag the music with. So you could imagine you have a track,
you feed it into our system and our system will spit out your metadata for it. It
is a rock track that is kind of in a happy uplifting mood. It contains the cars,
contains shakers, percussion and a female vocalist for instance. This is what you can
enrich your metadata catalogs with so that you can basically browse by certain tags.
The search itself consists of something that is actually a little more going into a
depth then more into an adept than the tagging itself so we are not searching for
certain keywords that appear in a search we really directly map text to music so we
make the system understand which kind of text description fits a song and for this
we use pairs of audios and text descriptions,
full text descriptions for the audio and we let our systems learn how much the
texts are actually related to the original audio and for that by this we allow our
AI to understand a some kind of a space where you can imagine any music or piece
in connected to any kind of text that you can type in.
The song you are listening to right now was found by Slipstream with the text
"Relax Sounds" for the background. Thirteen degrees is the song name and shrine the
artist's name.
Okay, sounds all easy. We just have to describe music and the models understand. But
it's not. Imagine a song like Bohemian Rhapsody, which we heard in the Whitney
Houston version earlier. You cannot label that song under one genre. Is it rock?
Is it opera? Is it pop? Songs have twists and turns and by describing them,
tagging them, one cannot say Minute 1 to 2 is rock, minute 3 to 5 is pop and in
between something else. It's too difficult. I'm also a music journalist now for
nearly 25 years. One thing I learned during that time, music is hard to describe.
I've read many critics of albums and each of them is different. Each of them
describes a song, an album differently. But that's okay. That is also the freedom of
music. Everyone can take something out of it, out of the songs, out of the album,
that fits best for him or her. An example, imagine you type in a text prompt like
generate me a song that is relaxing and reduces my stress. Some will say,
I connect this with heavy metal as it makes me happy and releases all my stress.
Others would argue, I need something that calms me down. Maybe some folk music.
Another example, a lot of people listen to sad music because it makes them happy.
They feel accompanied. Others can't listen to sad music as it makes them even more
sad. So yes, music tagging is not easy. In music what we kind of struggle with
often is especially when we compare it to the image domain where also I think a
lot of uprise has been happening within the last years that there's just a vast
amount of material available online, where the big companies simply scrape a lot of
pictures aligned with text description for it and build up Dolly with the mid
-journey and so on. This is not so easy for music because music is just a way
smaller field and it's also very data heavy, it's not only an image,
it's the whole audio file, so also harder to train the models on it because it's
just much more data heavy, the whole problem. And also finally we arrived to the
point that often with music it's not so clear what the music is actually about.
So if you imagine an image where you see a dog and a ball it's quite obvious that
everyone would say yeah this is a dog and a ball no one would say yeah maybe it's
a dog but it could also be a cat or something like this is kind of clear and
when we are talking about music we have very different ways to describe genres of
course there is somehow a certainty which direction it goes but there's very vast
amount of potential descriptions what you could call a track and it is even more
this for an emotion that is involved through music where there is of course a lot
of different words that you can describe it as but also the perception can be very
different from person to person so for one person it might sound like this for the
other person who might sound like this so it is way more a subjective thing than
with images which make kind of hard to actually gather metadata that is also
meaningful in a whole sense that could lead to an objective judgment by an AI
system.
You are listening to another song with a text search "relaxed sounds" for the And
the song is ironically called "This is the sign you've been looking for".
Credits go to the artist called Ringo. Let's come back to datasets. Music comes from
different parts of the world and a model might understand the music of one part of
the world, but that does not mean at all the music from the other part of the
world. The model has understood the structure of one catalogue, which might be very
western -focused rock music or something like this and then you apply it to some non
-western music and it absolutely doesn't work. What is actually also a very big
problem in our field that western music is just way more available in the internet,
especially there's a lot more metadata for it available and it's not so much the
case for music that it's also out there and also important but it's not like so
much focus on what we are usually hearing in our spheres and therefore it just
needs a very good curation and bringing together different data sources also taking
care of the data quality where we go through different very I would say in -depth
analysis for actually also guaranteeing the quality of a catalog and then,
yeah, step -to -step process, often in AI you do something,
you see how it works, you see where the problems are and then you think about how
can I solve it and how can I make it more accurate. It's really a back -and -forth
developing process that we are under. Not like you have an idea, you build one
thing and and it's there, it's always, you build, you refine, you refine, you
refine, because you also understand your data by the models that you train. So you
have a certain structure in the data which you might not even see, you train your
models on and the model has some kind of weird behavior. And out of that, you can
actually see what the data structure is like. And that is basically also a big Part
of the whole thing is also to understand music and to make some thoughts about what
is actually the stuff that you feed the AI with.
Nice dream, another song from Ringo, this time found under the text "Music to enjoy
the moment". All my text inputs sound maybe a bit silly, but hey,
in the end I was happy with the output. So the music was fine for this episode. I
hope you enjoyed episode 4 of the Iliac Suite and I could bring some light into
all these complex Questions concerning datasets?
Thanks for listening. Thanks Paul. Score down from Mubad and Roman Gipphardt from
Cyanide for talking to me. Take care and behave.
(upbeat music)
Creators and Guests
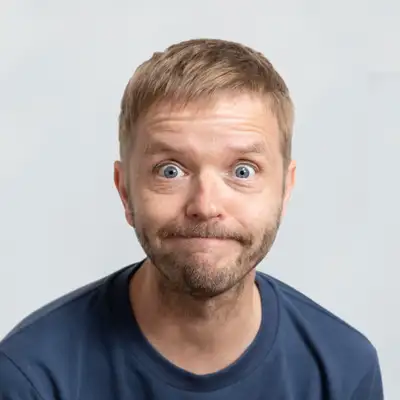
